Kowndinya Renduchintala
Research Associate 2, Media and Data Science Research (MDSR) Lab, Adobe Inc.
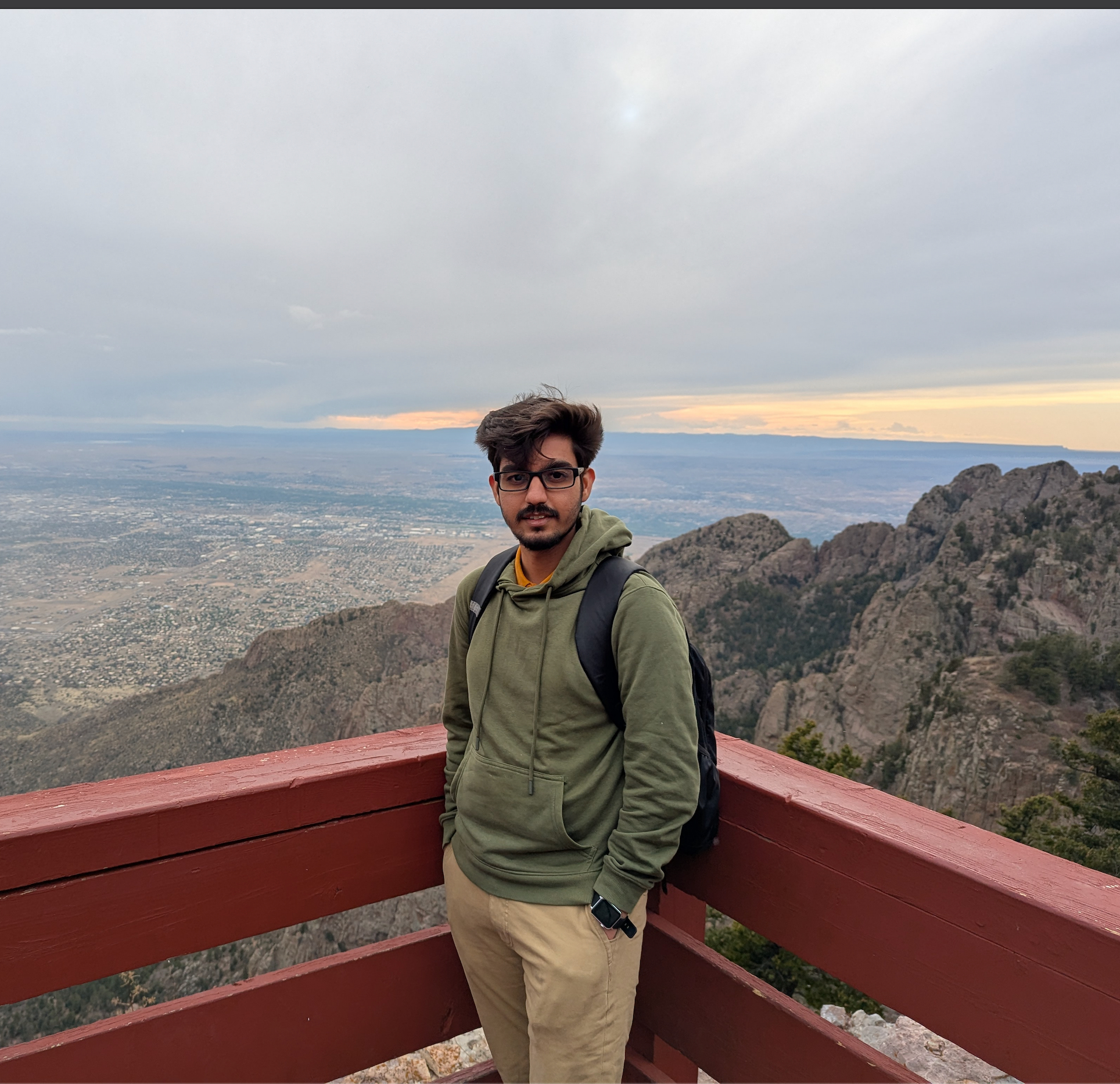
I am a Research Associate at Adobe, in the Media and Data Science Research (MDSR) lab where I am currently looking into various things like alternative loss functions for instruction tuning, coming up with novel pre-training procedures for LMs inspired from inductive biases that enable humans to acquire language so efficiently, and also scaling submodular optimization techniques to web-scale data.
Prior to joining Adobe, I graduated from the Indian Institute of Technology Bombay (IIT Bombay) in 2023, with a Bachelors degree (with Honors) in Computer Science and Engineering. I feel incredibly privileged to have been advised by Prof. Ganesh Ramakrishnan and Prof. Rishabh Iyer for my Undergraduate Thesis on Data-Efficient Pretraining of Language models using Submodular Functions, which I presented at EMNLP 2023.
My long-term research goal is to develop provably beneficial intelligent computational agents that can excel at both formal linguistic competence (the knowledge of rules and statistical regularities of language) and functional linguistic competence (the ability to use language in real-world situations).
If you share an interest in these topics and would like to exchange research ideas, please do not hesitate to contact me via e-mail.
news
Apr, 2025 | Attending NAACL 2025 in Albuquerque, New Mexico! ![]() |
---|---|
Nov, 2024 | Couldn’t present our work (in-person) at EMNLP 2024 in Miami, due to delays in visa processing ![]() |
Sep, 2024 | Our work on measuring prompt sensitivity of Language Models has been accepted to EMNLP 2024 (Findings)! |
Aug, 2024 | Presented our work (in-person) on submodular data mixtures for instruction tuning, at ACL 2024, in Bangkok ![]() |
Jul, 2024 | Got promoted to Research Associate 2 |
May, 2024 | Our work on submodular data mixtures for instruction tuning has been accepted to ACL 2024 (Findings) |
Dec, 2023 | Presented our work (in-person) on Data-Efficient Pretraining of Language Models at EMNLP 2023, in Singapore ![]() |